[ad_1]
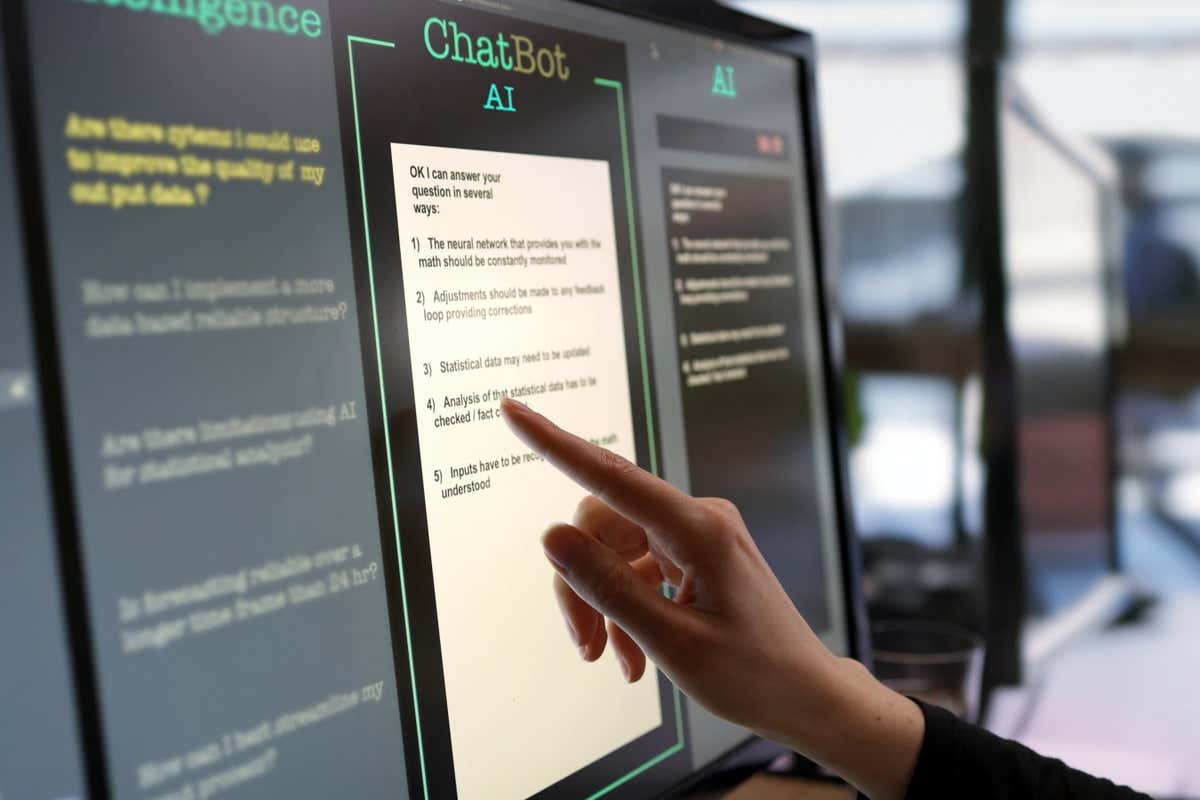
Chatbots use statistical models of human language to predict what words should come next
Laurence Dutton/Getty Images
Artificial intelligences that are trained using text and images from other AIs, which have themselves been trained on AI outputs, could eventually become functionally useless.
AIs such as ChatGPT, known as large language models (LLMs), use vast repositories of human-written text from the internet to create a statistical model of human language, so that they can predict which words are most likely to come next in a sentence. Since they have been available, the internet has become awash with AI-generated text, but the effect this will have on future AIs is unclear.
Now, Ilia Shumailov at the University of Oxford and his colleagues have found that AI models trained using the outputs of other AIs become heavily biased, overly simple and disconnected from reality – a problem they call model collapse.
This failure happens because of the way that AI models statistically represent text. An AI that sees a phrase or sentence many times will be likely to repeat this phrase in an output, and less likely to produce something it has rarely seen. When new models are then trained on text from other AIs, they see only a small fraction of the original AI’s possible outputs. This subset is unlikely to contain rarer outputs and so the new AI won’t factor them into its own possible outputs.
The model also has no way of telling whether the AI-generated text it sees corresponds to reality, which could introduce even more misinformation than current models.
A lack of sufficiently diverse training data is compounded by deficiencies in the models themselves and the way they are trained, which don’t always perfectly represent the underlying data in the first place. Shumailov and his team showed that this results in model collapse for a variety of different AI models. “As this process is repeating, ultimately we are converging into this state of madness where it’s just errors, errors and errors, and the magnitude of errors are much higher than anything else,” says Shumailov.
How quickly this process happens depends on the amount of AI-generated content in an AI’s training data and what kind of model it uses, but all models exposed to AI data appear to collapse eventually.
The only way to get around this would be to label and exclude the AI-generated outputs, says Shumailov. But this is impossible to do reliably, unless you own an interface where humans are known to enter text, such as Google or OpenAI’s ChatGPT interface — a dynamic that could entrench the already significant financial and computational advantages of big tech companies.
Some of the errors might be mitigated by instructing AIs to give preference to training data from before AI content flooded the web, says Vinu Sadasivan at the University of Maryland.
It is also possible that humans won’t post AI content to the internet without editing it themselves first, says Florian Tramèr at the Swiss Federal Institute of Technology in Zurich. “Even if the LLM in itself is biased in some ways, the human prompting and filtering process might mitigate this to make the final outputs be closer to the original human bias,” he says.
Topics:
[ad_2]
Source link